Machine learning/statistical methods in cancer research
Our focus in cancer epidemiology lies in leveraging the potential of machine learning methodologies. Machine learning offers a promising avenue to unravel complex patterns and relationships within cancer data. The integration of advanced computational techniques with complex cancer data allows for a nuanced understanding of cancer dynamics, risk factors, and the interplay of diverse variables. Through machine learning algorithms, we aim to discern intricate patterns in large datasets, identifying novel biomarkers, elucidating predictive models, and optimizing interventions.
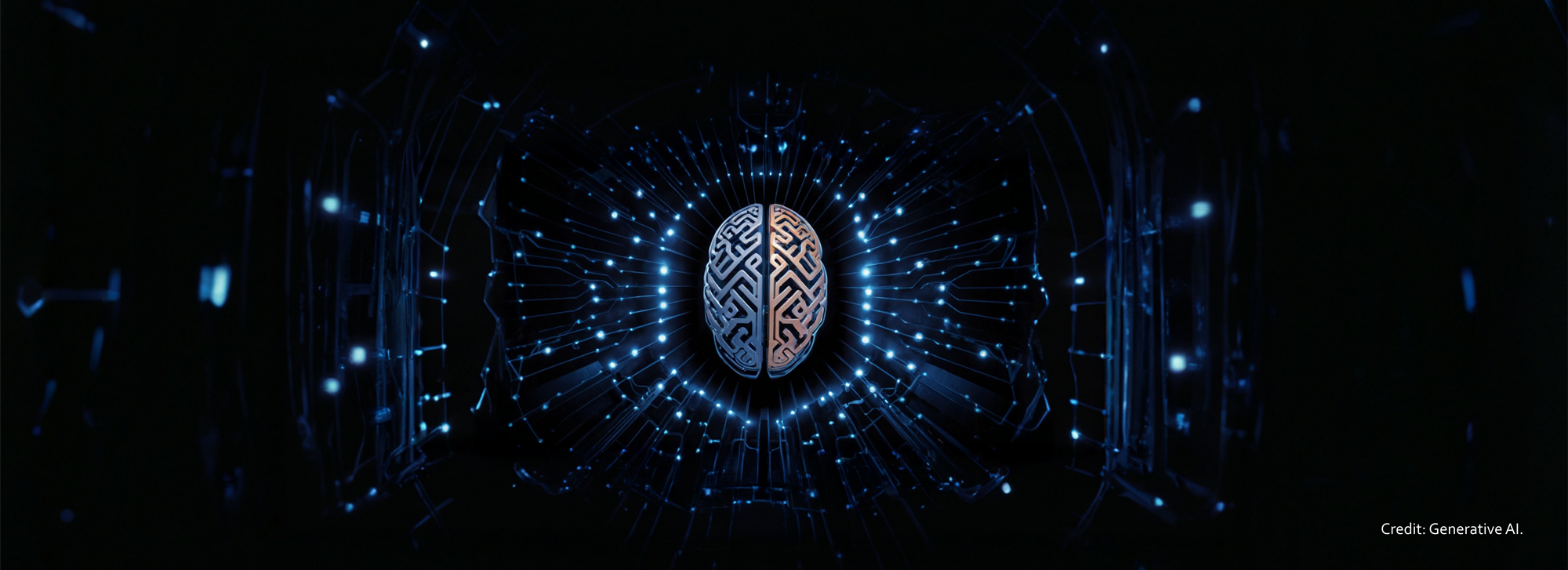
Publications:
Lung cancer:
- Machine learning-based immune phenotypes correlate with STK11/KEAP1 co-mutations and prognosis in resectable NSCLC: a sub-study of the TNM-I trial. Link to publication.
- A Pragmatic Machine Learning Approach to Quantify Tumor-Infiltrating Lymphocytes in Whole Slide Images. Link to publication.
- Artificial intelligence algorithm developed to predict immune checkpoint inhibitors efficacy in non–small-cell lung cancer. Link to abstract.
- Integrative, multi-omics, analysis of blood samples improves model predictions: applications to cancer. Link to publication.
Skin cancer:
- Recent advances in hyperspectral imaging for melanoma detection. Link to publication.
Breast cancer:
- A Probabilistic Bag-to-Class Approach to Multiple-Instance Learning. Link to publication.
- Publicly available datasets of breast histopathology H&E whole-slide images: A scoping review. Link to preprint publication.
- Cancer detection for white urban Americans. Link to abstract.
Other publications:
- Woes of The Practicing Omics Researcher. Link to book chapter.
- What is the state of the art? Accounting for multiplicity in machine learning benchmark performance. Link to preprint publication.
- Using machine learning methods to predict all-cause somatic hospital admissions and readmissions in adults: A systematic review. Link to preprint publication.
- Pizza made healthy – an epidemiological cookbook. Link to abstract.
- Facilitating the spread of cancer (data). Link to abstract.
- Dynamic detection of unexpressed genes in microarray mRNA. Link to abstract.