What causes an AI program to treat genders differently?
Artificial intelligence tends to perform worse on women, but why? What can be done to ensure that AI-related technologies work well for everyone?
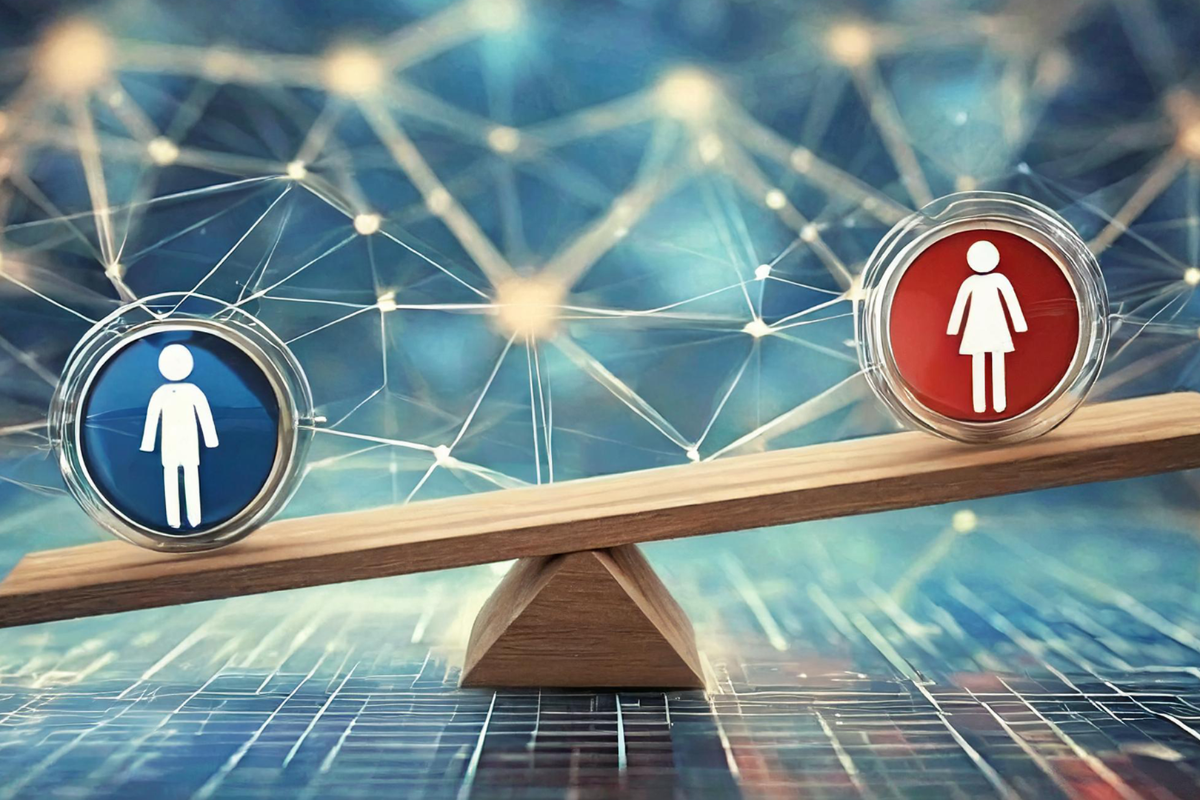
There is little doubt that artificial intelligence (AI) continues to show great potential in helping us in various areas, such as providing quicker medical treatment or streamlining time-consuming tasks at the workplace.
In order for AI technology to be helpful to society, it is important that such tools work well for everyone, regardless of gender, ethnicity, or sexual orientation. However, this is an aspect in which the technology tends to fall short of, say AI researchers at UiT The Arctic University of Norway.
– AI models have often performed less accurately on female populations compared to male populations, says PhD candidate Suaiba Salahuddin. She is, along with PhD candidate Srishti Gautam and associate professor Elisabeth Wetzer, a part of the UiT Machine Learning Group and the Center for Research-based Innovation, Visual Intelligence.
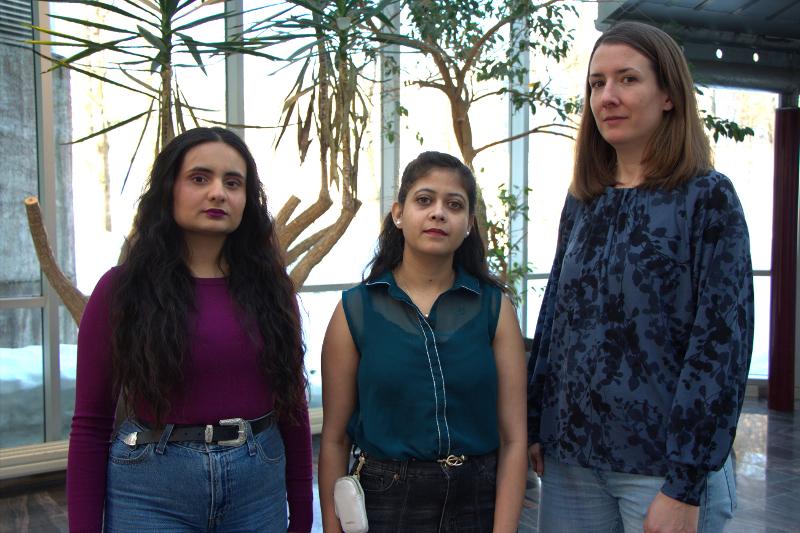
The researchers use Amazon's AI-based hiring tool as an example. The tool, which was supposed to streamline hiring processes at the company, quickly proved to favor applications written by men over women.
Large research projects like "Gender Shades" have also found that facial recognition tools from tech giants like Microsoft, IBM, and Face++ recognize fewer female faces compared to male ones.
These issues may undermine people’s trust in AI technologies.
– Such issues also pose a barrier to their widespread adoption, Gautam explains.
But what makes an AI system treat people differently based on gender?
Bias in data
How an AI system chooses to behave depends on the large amounts of data, which can be in the form of images, video, or text, it is trained on. The dataset, which it uses to identify possible correlations and patterns, is always ingrained in the AI’s “brain” as it performs a given task.
Such "big data" can represent different subgroups, such as people of different genders or skin tones. Which groups are represented in the training data, and to what extent, has a significant impact on which sub-populations the AI program works better or worse on, the researchers explain.
– The selection of data that is used to train a model and how it was collected may over or under-represent individuals from specific groups, such as gender identity. This ”bad” data can then influence the training of AI algorithms negatively, producing biased and even discriminative decisions, Salahuddin explains.
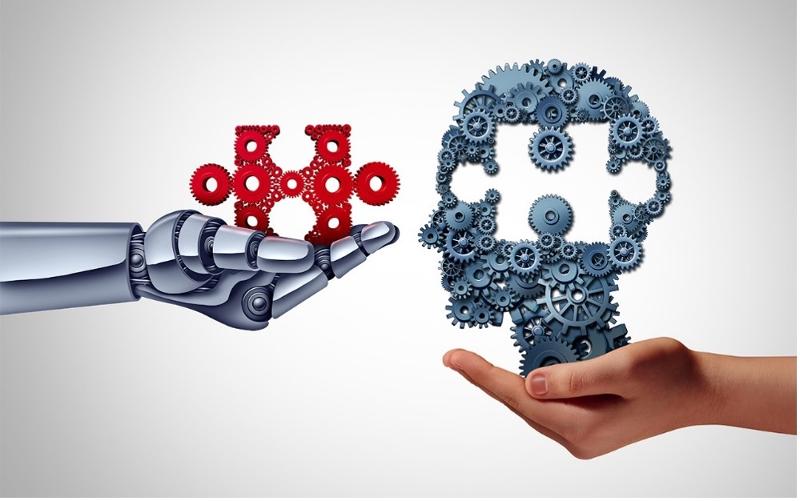
Data are also "historical," which means they reflect the reality from the time they come from. They may often be several decades old, thus reflecting gender-related biases at particular points in time.
Historical data such as salary statistics may, for example, show that women have, on average, lower income compared to men. If an algorithm is trained with this data, it may quickly assume that earnings are correlated with gender, and use this bias to determine who should be approved for a loan application or not.
– With such data, the model will likely reject more applications from women than men, Wetzer mentions.
It is therefore an important rule that AI is developed in such a way that it does not emphasize gender-related features. Even so, the programs are quite adept at picking up gender-related factors that a developer may not have realized exists in the data.
– For instance, the AI tool from Amazon learned to screen out resumes which contained the word ”woman” or mentioned women-associated colleges, Salahuddin explains.
Mute “black boxes”
Another challenge with AI is that such systems often do not explain the decisions they make, thus acting as inexplicable "black boxes".
As data are large, complex, and may contain biases that one may not have thought about, this "inexplicability" can make it challenging for developers to fully understand why an AI program chooses to treat genders differently, as well as make necessary corrections to the model.
So what can be done to prevent AI from using potential biases in the data when performing their tasks?
Developing AI models that can explain the reasons behind their decisions is an important goal within the field, and is something AI researchers at UiT are actively working on as a means for combatting the bias problem in AI.
This has been investigated by Gautam in her doctoral thesis "Towards Interpretable, Trustworthy and Reliable AI," which she submitted in December last year. As the title suggests, Gautam proposed and developed novel AI methods that can assist in making AI technology less susceptible to inheriting and using potential biases in their data.
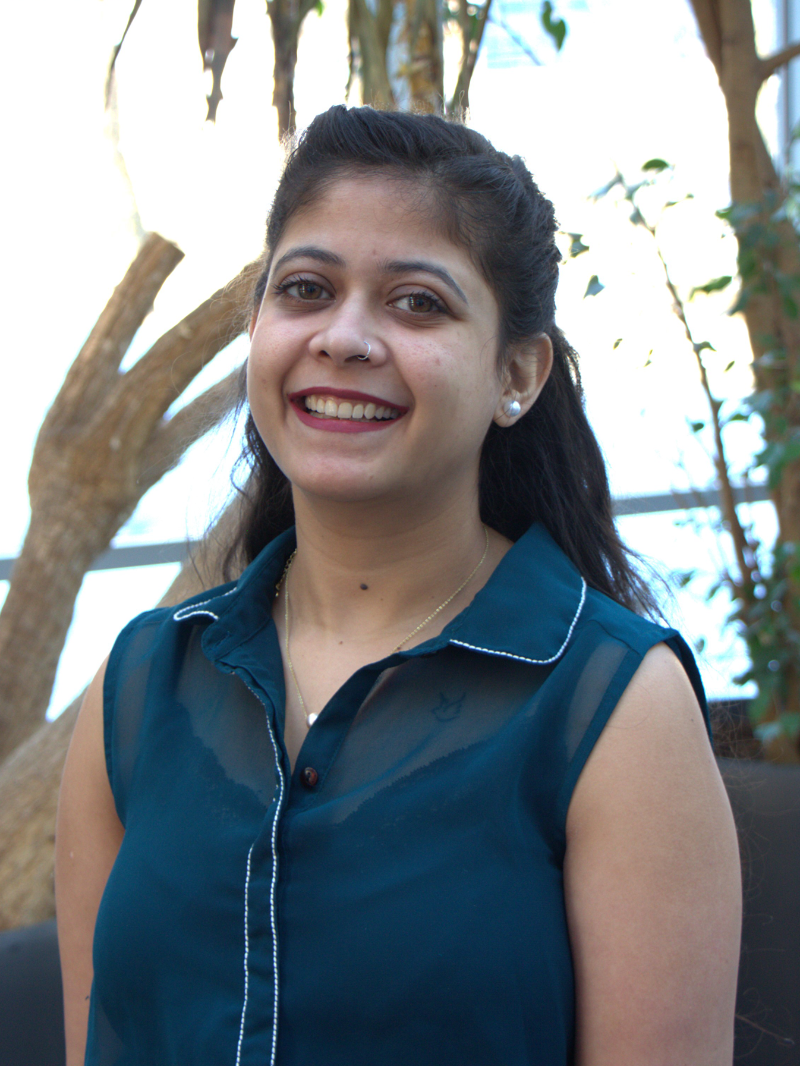
One of these is the "KMEx method": a method which can make the often mute "black boxes" "explain" what elements in the training data they have focused on when performing their task - without the need for retraining the model.
The benefits of "self-explainable" models are significant. If the AI program can explain its decisions, for instance why it produces different results for women and men, it becomes easier for developers to understand what the AI is focusing on in the data.
– Adopting more "explainable" or "transparent" AI models can significantly enhance safety in critical scenarios. For instance, in cases like the Amazon recruiting model, if the model offers explanations for its decisions, such as the reasons behind a CV's rejection, it becomes easier to identify and address any underlying biases, Gautam tells.
Results from her thesis, in which “KMEx” was tested on seven different sets of image data, show that the method can be a promising tool for making these "black box" algorithms more self-explanatory.
– Marginalized and underrepresented groups stand to benefit from efforts to reduce biases in AI, aiming to ensure equitable applications and prevent the perpetuation of social inequalities, Gautam states.
Lack of diversity in the field
However, the bias problem in AI extends beyond just data. Men comprise around 70 % of those who work on AI development globally, meaning that AI-based solutions are often designed and developed by this single group.
AI programs developed by a singular group often risk forgetting to consider other groups’, such as minorities’, views on and experiences with the technology. Such AI solutions will then be based on how that homogenous group understands, experiences, and interprets the world.
– When AI systems are predominantly designed by a homogenous group, they are more prone to embed and perpetuate existing biases, potentially leading to unfair outcomes especially for underrepresented groups, Gautam mentions.
– Without incorporating diverse perspectives and experiences, it is unlikely to achieve unbiased AI decision systems, Salahuddin adds.
It is in other words important that the research field reflects the diversity of the population. Increased focus on diversity and inclusion of AI researchers of different genders, ethnicities, and sexual orientations is therefore essential for creating fair, diverse, and inclusive AI solutions.
– The field needs as many perspectives as possible such that minority voices are heard, Wetzer mentions.
However, it requires a wide range of measures to ensure a more inclusive agenda in the field.
– We need role models. The lack of diverse role models in the field is a key contributor for the lack of diversity among students, researchers, and engineers further down the line, Wetzer states.
– We need to shed light on existing leaders in the field who come from a diverse set of background, so that youths have someone to identify with and be inspired and motivated by, she adds.
– Other measures may include creating educational programs that encourage diverse participation from an early age, actively recruiting and retaining a more diverse workforce, and fostering an inclusive environment where all employees can thrive, Salahuddin adds.
The necessity of regulation
AI technology is also evolving rapidly, which has led to discussion on how the development of AI technologies should be regulated. There is currently no explicit AI law in Norway.
Fortunately, the EU countries are working tirelessly to make such legislation, which will have a significant impact on how a potential Norwegian AI law will take shape.
Such regulatory guidelines will be an important step in combatting bias in AI, which are warmly welcomed by the researchers.
– To address the issue of data bias, it is essential to implement regulations that ensure the development of AI technologies is guided by ethical and inclusive principles, Gautam concludes.
Visual Intelligence
Visual Intelligence is a Center for Research-based Innovation that is led by the UiT Machine Learning Group. The center consists of a consortium of corporate and public user partners from different business areas, such as The University Hospital of North Norway, The Cancer Registry of Norway, Helse Nord IKT, Kongsberg Satellite Services and the Norwegian Computing Center.
-
Fiskeri- og havbruksvitenskap - bachelor
Varighet: 3 År -
Fiskeri- og havbruksvitenskap - master
Varighet: 2 År -
Akvamedisin - master
Varighet: 5 År -
Bioteknologi - bachelor
Varighet: 3 År -
Master of Philosophy in Visual and Multimodal Anthropology
Varighet: 2 År -
Sosialantropologi - master
Varighet: 2 År -
Arkeologi - master
Varighet: 2 År -
Musikkteknologi
Varighet: 2 Semestre -
Peace and Conflict Transformation - master
Varighet: 2 År -
Indigenous Studies - master
Varighet: 2 År -
Computer Science - master
Varighet: 2 År -
Human Rights Policy and Practice - master
Varighet: 2 År -
Geosciences - master
Varighet: 2 År -
Technology and Safety in the High North - master
Varighet: 2 År -
Physics - master
Varighet: 2 År -
Mathematical Sciences - master
Varighet: 2 År -
Molecular Sciences - master
Varighet: 2 År -
Sosiologi - master
Varighet: 2 År -
Strategisk ledelse og økonomi - erfaringsbasert master
Varighet: 5 Semestre -
Luftfartsfag - bachelor
Varighet: 3 År -
Samfunnsplanlegging og kulturforståelse - bachelor
Varighet: 3 År -
Sosiologi - bachelor
Varighet: 3 År -
Arkeologi - bachelor
Varighet: 3 År -
Spansk - årsstudium
Varighet: 1 År -
Statsvitenskap - bachelor
Varighet: 3 År -
Pedagogikk - master
Varighet: 2 År -
Informatikk, datamaskinsystemer - bachelor
Varighet: 3 År -
Informatikk, sivilingeniør - master
Varighet: 5 År -
Likestilling og kjønn - årsstudium
Varighet: 1 År -
Geologi - bachelor
Varighet: 3 År -
Samfunnsplanlegging og kulturforståelse - master
Varighet: 2 År -
Kjemi - bachelor
Varighet: 3 År -
Økonomi og administrasjon - bachelor
Varighet: 3 År -
Samfunnssikkerhet og miljø - bachelor
Varighet: 3 År -
Økonomi og administrasjon, siviløkonom - master
Varighet: 2 År -
Ledelse, innovasjon og marked - bachelor
Varighet: 3 År -
Ergoterapi - bachelor
Varighet: 3 År -
Grunnskolelærerutdanning for 1.-7. trinn - master
Varighet: 5 År -
Grunnskolelærerutdanning for 5.-10. trinn - master
Varighet: 5 År -
Automasjon, ingeniør - bachelor (ordinær, y-vei)
Varighet: 3 År -
Samfunnssikkerhet - master
Varighet: 2 År -
Kunst - bachelor
Varighet: 3 År -
Kunsthistorie - master
Varighet: 2 År -
Musikkutøving - bachelor
Varighet: 4 År -
Farmasi - master
Varighet: 2 År -
Religionsvitenskap - årsstudium
Varighet: 1 År -
Russisk - årsstudium
Varighet: 1 År -
Romfysikk, sivilingeniør - master
Varighet: 5 År -
Klima og miljøovervåkning, sivilingeniør - master
Varighet: 5 År -
Samfunnsøkonomi med datavitenskap - bachelor
Varighet: 3 År