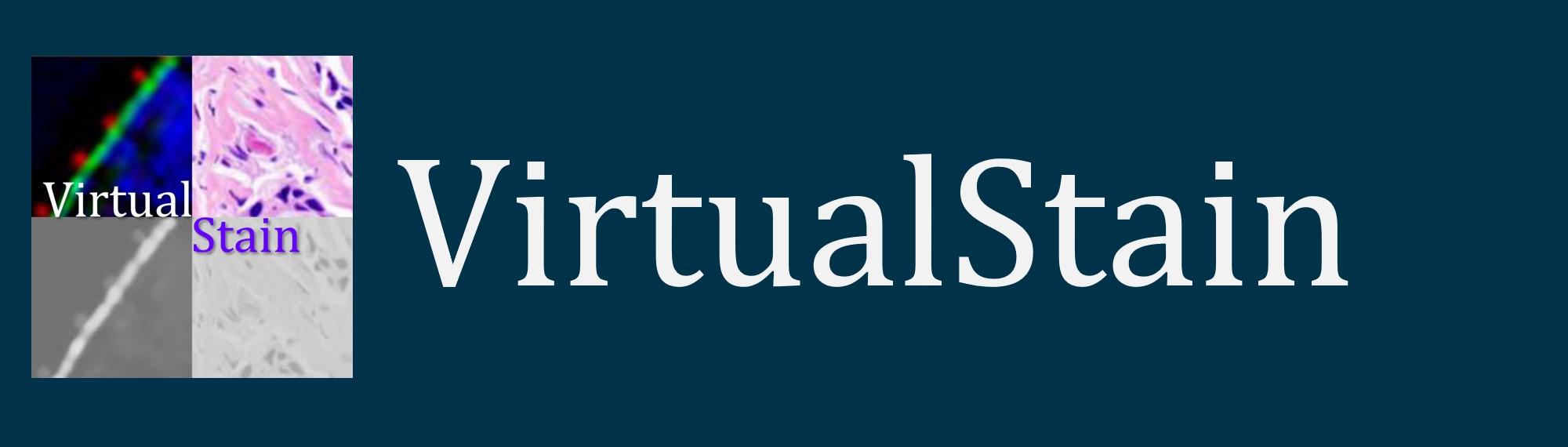
Background
Tissue and cell samples for medical research are typically treated, stained and imaged using microscopes. The dyes used for staining attach only to specific structures within the tissue or the cells, making them identifiable on the microscopy images. The staining procedure also have several short-comings. The noxious nature of the chemicals for fixing and staining the tissues and/or cells effectively freezes the tissue or cell in that specific moment, and cause the tissue/cell to die.
Only very few options exist for imaging living systems. These have many limitations in terms of resolution, penetration/optical sectioning, and are limited to 2D. Additionally, interpreting these label free images is challenging as they are grey-scale, with very few discernible structures.
Developing AI tools to process and label microscopy images of living tissues and cells has the potential to both obviate the demanding task of chemically staining tissues and cells, allow for simple and simultaneous labelling of multiple tissue/cell structures, and enable researchers to study active systems.
Doing this using conventional AI approaches would however require large training data-sets consisting of correlated sets of conventionally labelled images and label-free images. This can not easily be produced. Thus, new out-of-the-box approaches to developing AI solutions are required.